Transform to win: Moving from carbon budgets to decarbonisation action
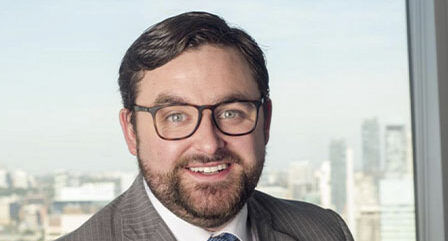
Carbon Budget 1, published in October 2021, allows us to generate up to 295 million tonnes of CO2 equivalent (MtCO2eq) of greenhouse gases over the five years from 2021 to 2025 inclusive. This calls for an annualised reduction in our emissions of approximately 4.8 per cent annually, write Stephen Prendiville, Head of Sustainability, and Anna Farrell, Sustainability Associate, of EY Ireland.
To put that reduction in context, during 2020, we achieved a 3.6 per cent reduction on our prior year emissions, due of course almost entirely to Covid-19 lockdowns. As such, Carbon Budget 1, for many, will demand significant changes to their lives.
And yet, 2021 has come and gone, and our emissions look set to be confirmed as having remained at 2019 levels. It means the changes of the next four years will be all the greater just to come within budget.
If this is going to be the narrative of our decarbonisation transformation, then our carbon budgets, which ought to be a very positive tool in our journey, could easily become a handicap.
It’s a fine line between a budget that motivates and budgets that are burdens. Feeling like you have some control in order to meet the budget is a key ingredient to ensuring motivation is maintained, but in general, budgets suffer right at the definition; they are a restriction.
Targets on the other hand, come with action orientation and motivation in the definition. Targets are meant to be achieved or even improved upon. Stretch targets will drive new thinking and innovation. Indeed, in business just meeting the minimum targets is not the mark of long-term success. We strive in everyday life to hit stretch goals, to innovate with a view to exceeding our expectations.
Our carbon budgets are effectively our minimum targets for decarbonising our economy. The minimum! Anyone will tell you, when you aim for minimum targets, you will invariably miss them, on the low side. It is why we set ourselves stretch goals. We cannot hope to unlock the opportunities and the value of this journey, in terms of jobs, skills, flows of capital etc., if our entire mindset is pointed towards minimum effort.
We need to be unshackled in our decarbonisation ambition. We need to start taking aggressive and positive actions right now. We need to transform to win.
What we need is a value-led approach and mindset. Value-led sustainability is a shift in mindset that looks at the opportunities presented by sustainability, rather than focusing on challenges. It’s engaging, and potentially investing, with one of your suppliers to decarbonise a product so that it creates opportunity for both. It is win-win focused. It’s creating a new sustainable product that opens you up to a new market and creates new revenue streams. It is looking at your processes and maximising how your waste products can be used differently or even recategorised as new products rather than “waste”. But more than anything, “value led” is action focused.
Soon, the Government will publish the allocation of sectoral carbon budgets. Sector representatives are currently, and I imagine will continue, to seek the maximum budgets for their particular groups – such that their groups will have the least burden in terms of decarbonisation. This siloed mindset will not help us. Rather we need cross sectoral collaboration to identify, and cross invest in decarbonisation pathways that help multiple sectors at a time. Taking a value-led approach, we might find that investments in technologies in one sector can have applications and benefits in another.
While debates continue back and forth over sectoral ceilings or the dilemma of energy decarbonisation, or the timing with respect to the strength of the national economy, the world continues to edge closer to disastrous climate change outcomes. This big picture continues to be lost while we are finalising perfect plans.
At EY, we have invested in not just meeting the minimum standards, but we’re striving to achieve as much as we can. We have committed to not just being carbon negative by 2021, which was achieved, but also to reducing total emissions by 40 per cent and achieving net zero in 2025. Setting the goals is one thing – taking action to self-disrupt your business and realise the value that it will create is quite another. We’re on this journey ourselves, so we understand the challenge faced by businesses in adapting a transformation and implementation mindset. It is from our own experience and in discussing these challenges with our clients, that we created EY Carbon, our end-to-end decarbonisation journey managed service solution that brings together expertise from across our firm to drive decarbonisation from the pages of the strategy right to action on the factory floor. Crucially, it’s not focused on creating the perfect plan.
Bold action is required, and we won’t find the answer in documents or excel sheets, we’ll find it in our communities, our businesses, and our families. The time for perfect planning has come to pass. We need to think about our decarbonisation targets and strive to exceed them, not just come within budgets.
E: sustainability@ie.ey.com
W: www.ey.com/ie/sustainability