Exploring common questions about choosing the right cloud infrastructure for AI workloads
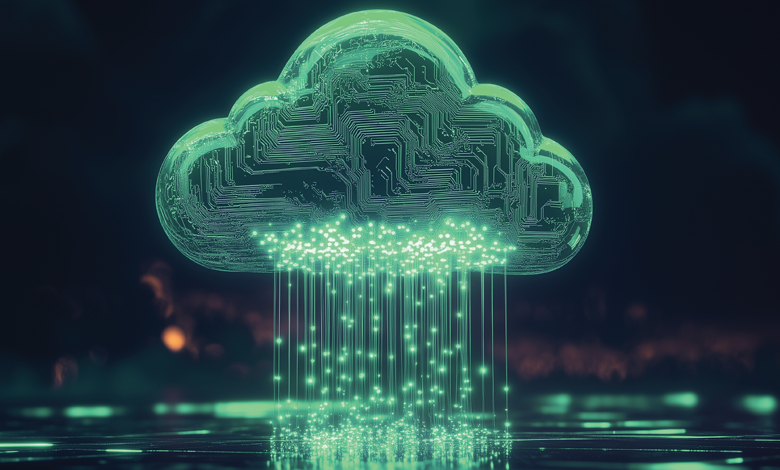
As AI continues to transform the enterprise landscape, a key decision looms: Should organisations rely on public cloud or private cloud to support their AI workloads? Rajesh Raheja Senior Vice President and General Manager for Private Cloud, HPE and Carita O’Leary, Sales Leader Ireland, HPE, write.
The answer to this fundamental question is increasingly clear. Hybrid cloud – balancing private cloud with selective use of public cloud – offers the best combination of performance, security, cost management. Some of the following are key questions in exploring what is the right fit for today noting the mix of workloads.
Q: How do you typically decide between public and private clouds and how does deploying AI change this approach?
Private cloud use for AI is seeing remarkable growth. Public cloud offers advantages like elasticity, ease of use, and quick access to new features. But many Users still prefer on-premises or private cloud solutions for a variety of reasons, such as security, governance, latency, and lower cost of ownership. The choice between public and private cloud depends largely on the enterprise’s workload needs.
AI is one of the workloads behind the resurgence of interest in private cloud. AI workloads require very large amounts of data and consistent utilisation of compute resources, this can be more cost effective on private cloud. Data gravity is also critical. High-quality data needs many complex data pipelines to feed and train machine learning models, it is more efficient and less costly to compute close to where the data resides, which may largely be on-premises. Therefore, users tend to adopt a hybrid cloud approach, blending both public and private cloud depending on workload requirements.
Q: With regulations like the EU’s General Data Protection Regulation (GDPR) and the EU AI Act in mind, how do public and private clouds compare in terms of data governance and sovereignty?
Private cloud provides ownership, hence, more control, governance, and flexibility over security policies. This is why it is increasingly preferred to meet regulatory needs such as GDPR, other data sovereignty, risk and control considerations under the EU AI Act also. Public cloud can handle some of these needs, but users may struggle with issues like data leakage, transparency in incident management, ensuring true isolation of resources and sensitive data.
Q: How do security requirements influence the choice between public and private clouds? Are there specific AI applications where one type of cloud is significantly more secure?
Security overlaps with regulatory, compliance concerns because a lot of the regulatory requirements, like FedRAMP, also tie into security. There is much to consider around data sensitivity, role-based access control, ensuring that proprietary data is locked down, including who holds the encryption keys – the cloud provider or the enterprise. Having full control in a private cloud gives customers much more assurance that their data is secure. While managing all this can be complex, managed private cloud services make it simpler for users to handle security without giving up control.
Q: How does the total cost of ownership of running AI workloads compare in public versus private clouds? Are there hidden costs to be aware of with either option?
Public cloud’s flexibility, elasticity come with a steep price. Public cloud costing may appear simple because for each CPU used per minute, you pay a few cents. This may work quite well for development teams experimenting before committing to any infrastructure. But as usage grows, especially with production AI workloads, costs can quickly escalate. At scale, public cloud bills can take up a significant portion of the IT budget, with hidden costs including storage costs, ingress charges due to moving data between regions.
There are also costs related to security, compliance customisations, which may come as a surprise. Owning resources outright can reduce costs significantly. According to recent data, private cloud can deliver 50 per cent lower TCO compared to public cloud.
It also provides 65 per cent higher workload performance, making it an attractive option for users that need high performance, predictable costs. With the rise of generative AI, we are going to see a mix of approaches when it comes to where compute resides.” Although public cloud is beneficial for quickly testing new technologies on a small scale, private cloud can offer better long-term value for sustained AI workloads, especially when security, regulatory, performance factors are considered. This is why CIOs are now being more thoughtful in their choice of where workloads are executed.
Choosing the right cloud infrastructure is crucial for managing AI workloads. Each option has its strengths, the best fit ultimately depends on your enterprise’s specific needs. Private cloud often provides the control, security, flexibility required for handling complex AI operations, while reducing the complexities of managing infrastructure. As AI initiatives grow, evaluating managed private cloud services could offer a solution that balances performance with operational efficiency, helping your business stay agile in a rapidly evolving landscape.
1 “You don’t have to be a data scientist,” Boeing
2 “The New 2023 Cloud Reality: A Rebalancing Between Private and Public,” HPE, June 15, 2023
3 Ibid About the author Rajesh Raheja is the senior vice president and general manager of the Private Cloud group within the Hybrid Cloud organization at HPE. He is responsible for leading the teams that develop the technology’s offerings, with a focus on expanding the Private Cloud portfolio. Article Extract from The Doppler.
W: www.hpe.com/