Data: The new oil
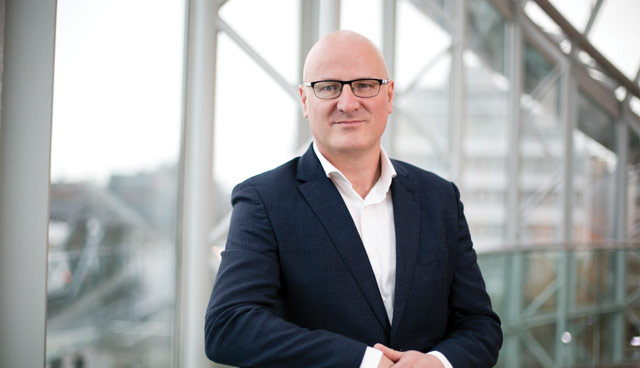
Data is the new oil and speed is now the currency in which we trade, writes Marc O’Regan, Dell Technologies CTO.
As the market continues to accelerate toward being more data-driven, it is becoming increasingly clear that what differentiates the most data-driven companies from the least data-driven, in terms of their ability to convert adoption of emerging technologies into actionable business insight, is speed. Speed at many different levels and layers.
Of all the “emerging tech” trends (and there are many), one stands king supreme above all others as we close out the second decade of the 21st century. This is of course Artificial Intelligence (AI).
There are a number of trends that have been driving change in the AI space in recent years, not least of which being the emergence of data science and the practical use of algorithms in the machine learning and deep learning spaces, along with emerging data platforms that enable us to store and process data that was previously idle, stranded, ignored or all three. It is data science, the use of advanced mathematics and mathematical functions to prise open information from data that ultimately results in outcomes, blended with advancements in computer technology and our attitude toward and relationship with data that has indeed accelerated our world into the new era of AI.
Along with algorithms and advanced computation facilities, the accuracy of the models constituting AI rely heavily on the availability of real-world data and the speed at which we can access, cleanse and convert it into usable and valuable information.
In AI training, researchers strive to train their networks faster, but this can lead to a decrease in accuracy. As a result, training machine-learning models at high speed while maintaining accuracy and precision is a vital goal for data scientists.
So too is the architecture and the methodology used during the training process.
The time required to train AI machines is affected by both computing time and communication time. Adopting a simple computational method to replace the more traditional methods used previously makes computation much faster without losing accuracy. As communication time is affected by the size of data blocks, we are now looking at communication techniques that will combine smaller pieces of data into larger ones, optimising the transmission pattern and thereby improving the efficiency of communication during AI training.
Driving these new AI applications and workloads also requires a change and approach to the underlying engineering platform. Today, we see vast improvements in engineering architecture that is propelling the AI space forward, but we need more.
Deep neural networks must and will get larger and faster, both in multi-cloud environments and at the edge. This means energy-efficiency must improve dramatically. While better accelerator technologies such as GPUs, FPGAs and other digital platforms can help to some extent, such systems unavoidably spend a lot of time and energy moving data from memory to processing and back.
We can improve both speed and energy-efficiency by performing AI calculations in the analog domain at the location of the data. However, this only makes sense to do if the resulting neural networks are just as smart as those implemented with conventional digital hardware and of course, if the horsepower is available at the edge to support the model deployed.
Acceleration
We are working hard in industry to evolve new technologies that will drive significant change in these spaces also. For instance, new memory technology that delivers data retention without power, high speed and high endurance abilities are emerging and now being produced with very high yields. Dated memory types such as DRAM could be replaced with these modern innovations in as little as 24 months. Data will fuel the development of AI and the pace of all this change will continue to accelerate in 2019. It will also highlight the fact that these trends are actually influencing each other to drive that change.
There is also the ‘people and process’ element to be considered here. Reducing the friction between consumers and operators involved in running ML data pipelines is one of the core components of what we are calling “DataOps”, a movement that is growing rapidly as we look for more agile approaches to data management.
Distributed architectures are now emerging and converging with more traditional approaches to data processing. Technologies like Blockchain and Distributed Ledger Technology (DLT) are now providing a new choice in terms of data storage and processing architecture and are finding their way into the new AI ecosystem. It remains early stages for Blockchain adoption, and the landscape is fragmented, but the use cases are becoming clearer and are now giving rise to the valuable implementation of Blockchain Applications.
Blockchain is concerned with keeping accurate records, authentication, and execution while AI helps in making decisions, assessing and understanding certain patterns and datasets, ultimately engendering autonomous interaction. AI and Blockchain share several characteristics which are ensuring a seamless interaction between these two emerging tech giants and they are massively complementary.
Blockchain for instance, emphasises the importance of data sharing between multiple clients on a particular network. Similarly, AI relies greatly on big data, more so, data sharing. With more open data to analyse, the prediction and assessment of machines are considered more correct, and the algorithms generated are more reliable.
There is a real need for security when dealing with high-value transactions on the Blockchain network. This is enforced via the existing protocols. For AI, the autonomous nature of the machines also requires a high-level of security in order to reduce the probability of a catastrophic occurrence.
Also, there is no greater threat to the advancement of any widely-accepted technology than lack of trust and neither AI nor Blockchain are excluded. To facilitate machine to machine communication, there is an expected level of trust. To also execute certain transactions on the Blockchain network, trust is required.
“AI and Blockchain share several characteristics which are ensuring a seamless interaction between these two emerging tech giants and they are massively complementary.”
The most compelling use cases which I believe will greatly reduce inefficiencies and unlock value, are in areas of existing industry where trusted intermediaries are required to record, validate and reconcile transactions without really adding additional value to the original transaction. This can and will be achieved through their blended implementation of both of these technologies.
Recently AI has the availability of huge data sets to train systems and sufficiently powerful compute capacity to execute the algorithms, making it possible to exploit AI in an increasingly large set of use cases. Combined with incredible leaps in computing power, processing and cloud innovation, all this data makes possible the AI training and learning that delivers truly intelligent systems, apps and technologies.
We are now using AI to solve some of the most important and complex problems on our planet today. We cannot say that there is a universally agreed upon determination of which technologies are considered ‘emerging’. Artificial intelligence has been here since the early 1950’s and distributed architectures have been with us for decades. What we can say though, is that AI embracing technologies like robotics, machine learning, deep learning, neural networks, virtual reality and augmented reality supported and streamed through our ecosystem through cloud computing, is having and will continue to have a monumental impact on society all over the world.
These technologies, enabled by significant advances in software, will underpin the formation of new human-machine partnerships. The technologies in play over the next decade have the potential to solve some of the intractable problems that humanity has faced for so long, offer the opportunity to increase productivity such that all our basic needs are taken care of, and fundamentally reframe notions of what it means to be a person.
Whether or not these emerging technologies will realise these ambitious possibilities is uncertain. What is certain is that they will intersect and interact with powerful demographic, economic, and cultural forces to upend the conditions of everyday life and reshape how many live and work over the next 10 years and beyond.
Marc O’Regan
Dell Technologies CTO – Ireland
W: www.dell.ie