Leading with purpose in the age of augmented intelligence
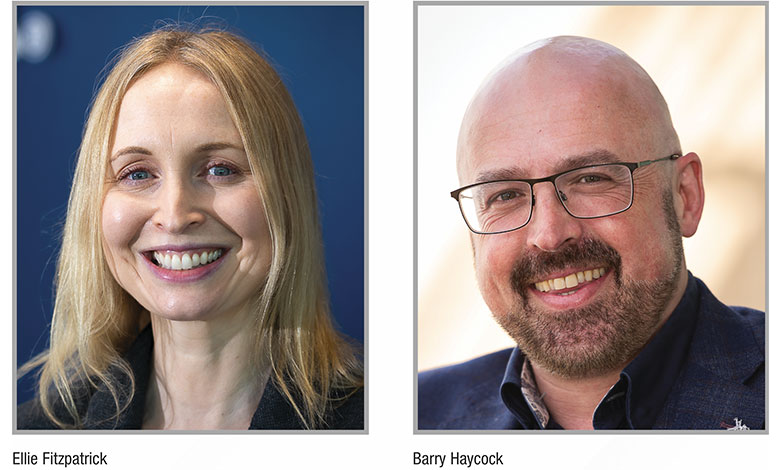
In today’s rapidly evolving technological landscape, artificial intelligence (AI) is transforming the way organisations operate, offering unprecedented opportunities for innovation and efficiency.
However, the journey to harnessing AI’s full potential presents challenges. Many organisations struggle to move beyond the ‘Proof-of-Concept’ stage, often due to a lack of strategic alignment between AI initiatives and overall business goals. Moreover, becoming the ‘Boss of AI’ also requires an understanding of which AI technology to use, often with a need to balance predictive and generative AI. But fundamentally decisions should be anchored to responsible and sustainable practices.
This article considers how prioritising strategic alignment and purpose, can turn AI into a powerful tool for sustainable innovation, ensuring long-term success.
Public sector organisations that do not strategically align their AI initiatives risk falling behind those who are leveraging AI as a force for sustainable innovation. According to Gartner, 60 per cent of AI projects will falter without strategic alignment to business goals. Strategic alignment of AI with business goals is non-negotiable for long-term success, requiring innovation, empowerment, ethics, and organisational transformation. For example, organisations with B-Corp accreditation are uniquely positioned to turn AI into a force for ethical growth.
Three areas are key to guiding your journey towards strategic, sustainable, and profitable AI utilisation. First, consider the various types of AI that can benefit your organisation. Second, focus on empowering your team to effectively utilise these tools while engaging in ethical considerations. Finally, emphasise the importance of governance to build trust in AI applications.
1. Types of AI: Leading with strategic clarity
By understanding the strengths and applications of different AI types, you can strategically implement solutions that enhance productivity and foster innovation.
For example, generative AI, like retrieval augmented generation (RAG), acts as a knowledge search engine, saving time by providing expert-like responses from internal documents. Customer-facing chatbots and AI video-call interfaces, such as BearingPoint’s Virtual Consultant, are also emerging in the modern, augmented workplace. Recently, BearingPoint implemented a RAG tool for the Department of Social Protection to help understand circulars and allow users to more easily navigate the directives prescribed within them. This effort saves time and reduces potential errors, helping people better address citizens’ needs. Generative AI used in this way is highly impactful, similarly, the Autorité des Marchés Financiers (AMF) in France is leveraging generative AI to enhance its supervisory functions, including pre-processing documents, detecting market abuse, and classifying ESG themes in issuer press releases.
Predictive AI, as a more mature technology, has had large-scale impact in fields like supply chain analysis, intelligent sourcing, etc. Unilever, for example, is using AI to locate sources of Palm Oil that do not contribute to deforestation. One example of this is in demand forecasting, within an Irish public sector department that has tight deadlines for delivery to citizens, we have built predictive tools that estimate upcoming demand and allow for staff to be assigned in time to meet demand, returning to other value adding work when demand will be lower.
Typically, strategic leaders use predictive AI for efficiency and GenAI for innovation. Through a balanced combination of both techniques, modern augmented workplace ideals can be reached in a way that is sustainable and ethically responsible while generating business buy-in.
2. Empowerment through ethical guardrails
The modern augmented workplace movement is also keenly focussed on upskilling teams, not replacing them. This makes sense on every dimension, people deliver their best when given the best tools for the job, and AI is just a new tool. With a view to B-Corp alignment, initiatives like AstraZeneca’s GenAI accreditation programme is a great example of empowering people. By gamifying training, employees not only learn technical skills but also gain confidence in integrating GenAI into workflows ethically.
Sustainability requires us to know how new initiatives will impact employees, but also the subjects of the predictions or analysis. There are now readily available tools designed to ensure that data bias and AI bias can be reduced effectively.
Tools like Microsoft’s Responsible AI Dashboard and Amazon’s Sagemaker Clarify, monitor AI predictions for bias and fairness. Explainable AI techniques, now widely adopted, allow us to understand the metrics behind individual AI decisions, exposing and mitigating latent biases.
The identification and mitigation of bias is an ongoing concern at academic and business level. Stanford University’s recent paper, Fairness through Difference Awareness: Measuring Desired Group Discrimination in LLMs proposes benchmarks to assess AI models’ awareness of biases. Thought leadership like this informs offerings like BearingPoint’s Data Strategy Framework to better enable wide adoption.
3. Governance: The linchpin of trust
Effective governance that simultaneously enables the benefits of AI while providing guardrails and enabling understanding of why the AI does what it does is critical for success in the future of work. This is achieved by building trust via the cross-functional leadership that enables AI in the first place. Implementing a framework that proactively addresses regulatory compliance (e.g., EU AI Act) while integrating sustainability metrics into decision-making processes is key.
Examples include how Pfizer’s cross-functional AI council oversees ethical AI deployment, while AWS uses AI to reduce data centre emissions. Aligning AI investment with employee goals maximises success, as shown in BearingPoint’s 2024 survey of 700 global companies titled Transitioning into an Augmented Organisation, where it is shown that leaders are more likely to include employees in AI decision making.
A responsible AI framework integrates principles like algorithmic fairness and transparency while minimising environmental impact through energy-efficient models. As the field evolves, both in policy and technical advancements, a sensible framework must continue to develop.
Our framework also includes privacy preserving methods, for example techniques like federated learning ensure data privacy by keeping sensitive information decentralised during model training.
Conclusion
The future belongs to organisations where AI serves purpose by delivering measurable value to citizens, communities, and the environment. Strategic alignment of AI initiatives with organisational goals is most impactful when it enables measurable improvements in citizen well-being, community resilience, and environmental sustainability. Invest in the right technologies, upskilling and practices for your needs that balance growth with environmental responsibility. Most importantly, build trust through transparency and ethical governance. Organisations that audit their initiatives against KPIs and ESG goals, adapting frameworks such as B-Corp’s Impact Assessment for public sector needs, will be more successful in implementing AI.
Organisations that align AI with purpose will thrive in an era where technology must serve humanity and sustainability.
W: www.bearingpoint.com