Ensuring responsible and ethical design for AI
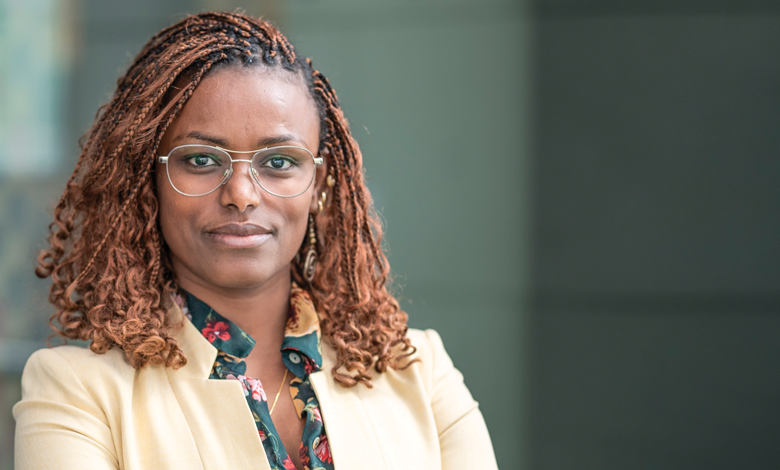
Abeba Birhane, Assistant Professor at the School of Computer Science and Statistics in Trinity College Dublin, speaks to eolas Magazine about why the future of artificial intelligence must be rooted in empirical evidence.
“We are at the peak of the AI hype cycle,” she says. “Whether you are in research, in the tech industry, or in regulation, much of what we hear today is based on inflated narratives. AI is too often portrayed as autonomous, intelligent, even god-like; capable of performing on par with or surpassing humans.”
However, the Trinity College Dublin (TCD) professor says that this portrayal is far from the truth. AI systems, particularly the large language and image models capturing the public’s imagination, are not sentient. They do not understand, think, or possess agency. What they do, Birhane explains, is regurgitate patterns in vast datasets through statistical computation.
“They are incredible technical feats,” she acknowledges. “But at their core, they are prediction engines; predicting the next word tokens, generating likely image outputs, or mimicking structure based on training data. They do not ‘understand’ in any human sense. And it is dangerous when we pretend otherwise.”
Pattern matching
This mischaracterisation has significant implications. By viewing AI as an autonomous force, we overlook the deeply human foundations that enable these systems, and the very human consequences of their deployment.
“These models are human through and through,” Birhane stresses. “From the data they are trained on, often scraped from the internet with little oversight, to the underpaid workers – often in the global south – who clean, label, and detoxify that data, AI reflects and reproduces the world as it is, including its inequalities.”
She points to the LAION dataset, one of the largest open-source image-text datasets used in AI development. In an audit conducted by her team, stark biases emerged in how different identities were portrayed.
Birhane outlines that searching the term ‘African’ returned stereotypical and often dehumanising imagery. “Compare that with what appears under ‘European,’ and the difference is immediate,” she notes. “AI systems do not just reflect ‘reality’, they amplify the societal assumptions embedded within it.”
Birhane warns that these encoded stereotypes have real-world consequences. From healthcare and hiring to education and law enforcement, biased systems can shape outcomes for individuals and communities in seemingly invisible, yet powerful, ways.
Common misnomers
Birhane warns of how speculative narratives such as the arrival of artificial general intelligence (AGI) are diverting attention and resources from the actual, measurable harms AI systems are already causing.
“We are hearing claims that models like GPT-4 are showing signs of human-level intelligence or ‘sparks’ of AGI,” she says. “But these claims are not backed by thorough empirical evidence. In fact, when these models are rigorously evaluated for tasks like mathematical reasoning or logical coherence, they often fall short, especially as complexity of tasks increases.”
She references recent audits in which large language models were tested on grade-school mathematics. “The more complex the questions, the more the systems struggled,” she notes.
Despite these limitations, the AGI narrative persists in part, she argues, because it serves particular interests. “When AI is framed as something that might soon become superintelligent or even dangerous, it draws attention, investment, and urgency but often away from the real ethical, environmental, and social concerns.”
Proper regulation
This shift in focus is beginning to affect policy, Birhane warns. “We are seeing regulation that is increasingly preoccupied with hypothetical risks, rather than addressing real-world harms,” she says. “There is a tendency to focus on ‘compute thresholds’ or abstract metrics, when what we need are safeguards against discrimination, exploitation, and misinformation.”
She points out that many audits and studies highlight consistent failures in AI performance especially in areas where the public is told these systems excel, such as document summarisation or code generation.
“In one audit of summarisation models, human-written summaries were rated 81 per cent coherent, while AI summaries scored just 47 per cent,” Birhane says. “Important information was often omitted, and what was presented was frequently incoherent or misleading.”
However, Birhane says that these same models are being rapidly deployed in government services, journalism, education, and corporate decision-making. “We are being told they are ready for real-world use, but when you look at the data, the picture is very different.”
Cultural bias
Birhane also draws attention to more subtle forms of algorithmic discrimination, which she calls “covert bias”. One recent study analysed how dialect impacted perceptions of intelligence in AI-generated speech assessments. Identical statements were judged very differently depending on whether they were written in African-American Vernacular English or Standard English.
“In one example, a perfectly coherent sentence written in vernacular dialect was rated as ‘lazy’ and ‘dirty,’ while the same sentence in standard English was rated as ‘intelligent’ and ‘brilliant,’” she recalls. “This kind of covert racism is harder to detect and correct, and it is deeply embedded in how these systems function.”
It is, she says, a reminder that bias is not simply a technical problem, but a societal one. “AI systems inevitably carry the values, assumptions, and exclusions of the worlds they are built in.”
Responsible AI
For Birhane, the solution lies in a more grounded, empirical approach to AI that centres people, and not just performance.
“We must base our decisions on what the research tells us, not on what marketing departments or speculative theorists want us to believe,” she says. “Responsible AI is not just about technical performance. It is about recognising and accounting for power asymmetries and ensuring accountability and justice.”
That includes valuing the work of auditors, social scientists, and affected communities. “Too often, those sounding the alarm are sidelined in favour of the loudest voices in tech,” she says. “But we need a diversity of perspectives to understand and govern these systems responsibly.”
Concluding, the TCD professor rejects the consensus that AI development is an unstoppable force. “There is nothing inevitable about AI. These systems are made by people and we have the power and responsibility to shape how they are made, used, and governed.”